Abstract
Web agents have emerged as a promising direction to automate Web task completion based on user instructions, significantly enhancing user experience. Recently, Web agents have evolved from traditional agents to Large Language Models (LLMs)-based Web agents. Despite their success, existing LLM-based Web agents overlook the importance of personalized data (e.g. user profiles and historical Web behaviors) in assisting the understanding of users' personalized instructions and executing customized actions.
To overcome the limitation, we first formulate the task of LLM-empowered personalized Web agents, which integrate personalized data and user instructions to personalize instruction comprehension and action execution. To address the absence of a comprehensive evaluation benchmark, we construct a Personalized Web Agent Benchmark (PersonalWAB), featuring user instructions, personalized user data, Web functions, and two evaluation paradigms across three personalized Web tasks. Moreover, we propose a Personalized User Memory-enhanced Alignment (PUMA) framework to adapt LLMs to the personalized Web agent task. PUMA utilizes a memory bank with a task-specific retrieval strategy to filter relevant historical Web behaviors. Based on the behaviors, PUMA then aligns LLMs for personalized action execution through fine-tuning and direct preference optimization. Extensive experiments validate the superiority of PUMA over existing Web agents on PersonalWAB.
PersonalWAB Benchmark
Our benchmark includes:
See more details in our paper: Large Language Models Empowered Personalized Web Agents.
Overall Pipeline
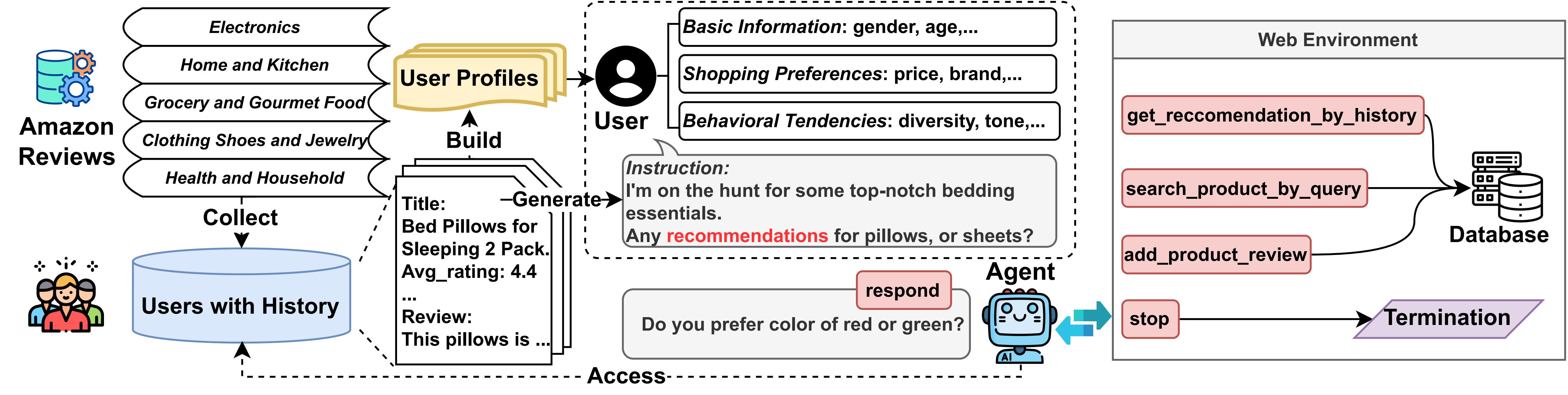